"But you didn't adjust for X!" Challenge Accepted.
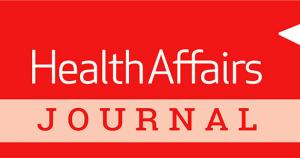
For over a year, I've been tracking the rates of both COVID-19 vaccinations as well as COVID-19 cases & deaths, broken out by county-level partisan lean (namely, what percent of the vote Donald Trump received in 2020).
I've received quite a bit of attention for these analyses, including several national media outlets which have used my work (sometimes with proper attribution, sometimes without) However, there have also been numerous critics who have pointed out that I don't run multivariate analysis when I do this.
Put simply, I look at the correlation between partisan lean and COVID death/vaccination rates or between vaccination rates and COVID death rates...but I don't include other factors like age, income, race/ethnicity, urban-rural status, employment status, health insurance status and so forth.
I did run vaccination rate analyses against population density, urban-rural status, education and income levels last year...but again, these were still done independently of any other variables.
The reason for this isn't because I'm trying to "hide" any of this data or because I feared it would debunk my theories; it's because, put simply, I have neither the statistical analysis skills nor the tools to run multivariate analyses properly.
Fortunately, there are others who do have both the skills and tools to do do...and in this month's issue of Health Affairs, they've done just that:
The Association Between COVID-19 Mortality And The County-Level Partisan Divide In The United States
Neil Jay Sehgal, Dahai Yue, Elle Pope, Ren Hao Wang, and Dylan H. Roby
The first four are from the University of Maryland; Roby is from the University of California Irvine.
Abstract
Partisan differences in attitudes toward the COVID-19 pandemic and toward the appropriateness of local policies requiring masks, social distancing, and vaccines are apparent in the United States. Previous research suggests that areas with a higher Republican vote share may experience more COVID-19 mortality, potentially as a consequence of these differences.
In this observational study that captured data from a majority of US counties, we compared the number of COVID-19 deaths through October 31, 2021, among counties with differing levels of Republican vote share, using 2020 presidential election returns to characterize county political affiliation.
Our analyses controlled for demographic characteristics and social determinants likely to influence COVID-19 transmission and outcomes using state fixed effects. We found a positive dose-response relationship between county-level Republican vote share and county-level COVID-19 mortality. Majority Republican counties experienced 72.9 additional deaths per 100,000 people relative to majority Democratic counties during the study period, and COVID-19 vaccine uptake explains approximately 10 percent of the difference. Our findings suggest that county-level voting behavior may act as a proxy for compliance with and support of public health measures that would protect residents from COVID-19.
And yes, they give me (and others who've done similar work) a shout-out, thanks!
...The authors thank the Centers for Disease Control and Prevention and the New York Times for facilitating access to county-level COVID-19 mortality data. They also thank Charles Gaba of ACASignups.net, David Leonhardt of the New York Times, Gregory Travis, and Michael Olesen for their work documenting the differences in vaccine uptake, COVID-19 mortality, and county voting records. Their work to elucidate these differences inspired the work presented here.
OK, so what variables did they take into account?
Covariates We included a range of county- level demographic and social determinants of health as covariates. We included urban-rural classification for counties from the CDC...
We also included the following county-level measures from the 2018 AHRQ Social Determinants of Health database: the proportion of the population who were female, who identified as African American, who identified as Hispanic, who did not speak English as a first language, and who were age sixty-five or older; the percentage of the population (age sixteen or older) that was unemployed; the percentage of the population with no health insurance and median household income; and the percentile overall ranking from the CDC’s Social Vulnerability Index, a census-derived measure that accounts for indicators of socioeconomic status, household composition and disability, minority status and language, and housing type and transportation (ranges from 0 to 1, with higher values indicating counties with greater vulnerability).
Moreover, we also included co-variates on county-level health resources in 2018 from the Area Health Resources Files.
These included the number of nurse practitioners, clinical nurse specialists, primary care physicians, and physician assistants per 10,000 people and the number of hospital beds per 10,000 people.
We included health personnel as proxies for health system capacity...
...county-level prevalence estimates for five health conditions with strong and consistent evidence of association with higher risk for severe COVID-19-associated illness. These include chronic obstructive pulmonary disease, heart conditions, diabetes mellitus, chronic kidney disease, and obesity. We examined the prevalence of any of these conditions in our analyses.
Finally, we included county vaccination rates.
Holy smokes. If you still don't think this corrects/adjusts for enough other factors, it's never going to be enough.
OK, so after correcting for all of these variables, what were their findings?
Compared with Democratic counties, the risk difference for Democratic-leaning counties was 5.78 but was not statistically significant. However, when compared with Democratic counties, the risk differences for Republican-leaning and Republican counties were 33.52 and 72.94, respectively, and were statistically significant. This model suggests that during the study period, the average cumulative number of COVID-19 deaths was higher by 72.94 deaths for every 100,000 people in Republican counties compared with Democratic counties when state-level factors, such as COVID-19-related policies, were also accounted for.
During the winter 2020–21 surge (September 2020–March 2021), COVID-19 mortality in Republican counties outpaced that in Democratic counties. Starting in April 2021 and continuing through the spring 2021 wave, Republican counties had a significantly higher COVID-19 death rate compared with Democratic counties. The difference widened through the end of the Delta variant surge (June–October 2021). A dose-response relationship was again apparent, with counties with the highest Republican vote share experiencing the highest risk difference in COVID-19 death rates, when all covariates included in the model were adjusted for.
More specifically:
There were 72.94 additional deaths per 100,000 people in majority Republican counties during our study period, and the difference cannot be fully explained by COVID-19 vaccination rates, according to our mediation analyses. This finding supports previous non-peer-reviewed bivariate correlation and descriptive analyses comparing death rates and vaccination rates by voting behavior.
By accounting for age, urban/rural status, vaccine uptake, chronic illness burden, race and ethnicity, health care availability, unemployment, and other factors in our model, we were able to address concerns that partisan differences in death rates were due to people living in areas with majority Republican vote share being older, less likely to have access to health care because of their rural location, or having higher underlying burdens of chronic disease. Instead, it appears that voting behavior acts as a proxy for compliance with and support for public health measures, vaccine uptake, and the likelihood of engaging in riskier behaviors (for example, unmasked social events and in-person dining) that could affect disease spread and mortality.
This study and their findings is even more significant when you consider that it includes the first full year of the pandemic as well...that is, unlike most of my analyses which started in May or June 2021 (since I've focused mostly on the post-vaccination era), this study includes the first wave of the pandemic which hit the blue/Dem-leaning counties extremely hard...yet even with all of that, they still come to pretty much the exact same conclusions as I have.
There's several tables, graphs, charts etc. in the study itself, but that's behind a paywall and I may have already used too much of it here, so I'll leave it at that.
To be clear: There was nothing wrong with pointing out that I didn't include the other potential covariates in my own work, and I'm glad to see that folks who are better at this sort of thing than I am have done the work to include them. WIth that being said, now that they've published their own findings, can we finally dispense with the "...but you didn't account for X!" defense? It's partisanship.
UPDATE: One aspect which I sort of skipped over when I first read their study is the "COVID-19 vaccine uptake explains approximately 10 percent of the difference" reference. Several people have commented that this is pretty surprising given how effective the vaccines are (in fact, my own tracking of death rates by vaccination level and partisan level finds the two to be nearly a mirror image of each other).
At first I shrugged this off because I thought that all of their findings included COVID deaths dating back to the beginning of the pandemic in March 2020; since well over half a million Americans had died of COVID by then, it made sense that vaccination rates would play a much smaller role in the total pandemic death patterns thorugh October 2021.
HOWEVER, I missed an important clarification, brought to my attention by one of the authors of the study:
While the main model showing differences in death rate is for all COVID deaths (pre- and post-vaccine), the mediation analysis that looks at the effect of vaccine uptake is limited to deaths that occurred after vaccines were available. Sorry if it’s confusing!
— Dylan Roby (@professor_roby) June 7, 2022
Sure enough:
Sensitivity Analyses We conducted several sensitivity analyses including the mediation effect of COVID-19 vaccination rates in the period after vaccines became widely available. We also assessed the robustness of our results to COVID- 19 mortality data from CDC and different model specifications (see "Other Analyses" in the appendix).
...Results from our sensitivity analyses provide further support for our main analyses. First, we found a statistically significant effect of higher county-level vaccination rates on reductions in COVID-19 mortality, as well as significant negative associations between Republican vote share and vaccination rates (appendix exhibit A4). Appendix exhibit A5 further shows that 9.98 percent (95% confidence interval: 0.08, 21.77) of the adjusted difference in COVID-19 death rates between Republican and Democratic counties was attributable to differences in vaccination rates.
They also note this as one of their limitations:
Finally, our study may have underestimated the effect of vaccination on COVID-19 mortality, as COVID-19 vaccines were not widely available until spring 2021 and vaccine effectiveness has differed over time because of changes in circulating SARS-CoV-2 variants.
From the appendix referenced:
First, we conducted a mediation analysis to explore whether the impact of voting differences on COVID-19 mortality is through county-level COVID-19 vaccination rate. Specifically, we obtained the total effect as marginal effect from the GLM adjusting for covariates. We then calculated the direct effect as the marginal effect from the GLM for covariates plus county-level vaccination rate. The indirect effect is defined as total effect minus direct effect. The percent of total effect mediated by the vaccination rate is defined as the ratio of indirect effect to the total effect. Since we used a non-linear model, standard errors for the indirect effect and ratio are not directly available from the model. We used bootstrapping with 1000 replications to compute the bias-corrected standard errors.
Again, I'm not enough of a professional statistician to interpret all of this, but it seems to me this is even more damning of other actions taken (or not taken) based on partisan leanings, like wearing masks, social distancing and so forth than I had figured. Huh.